Examples Of Bigdata & Machine Learning Use Cases In Industry 4.0, IoT, Banking
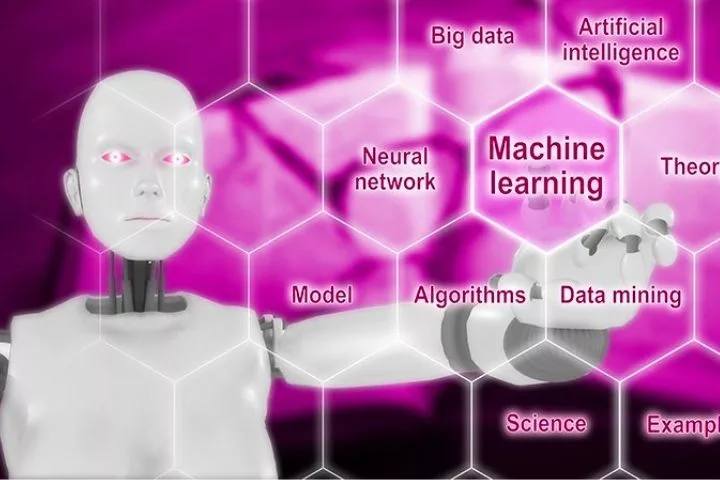
There is often a lack of examples of how technologies like artificial intelligence, data science, bigdata & machine learning can be implemented as use cases. We have therefore compiled a list of use cases for the area of AI and big data, which we are continuously expanding.
Bigdata & machine learning examples for companies
- Sales forecasting: The forecast of sales figures is one of the first use cases implemented in companies. On the other hand, however, it is one of the most difficult: seasonality, low data availability, highly volatile factors – all aspects that harm the quality of the models. Implementation mostly uses time series analysis.
- Automatic E-Mail Classification: Classification and pre-sorting of e-mails by assigning a recipient or a category. A major efficiency factor, especially in customer support, eliminates manual work, avoids errors and guarantees high speed.
- Process mining: Timestamps of certain process steps can draw a concrete picture of the actual process flow. This information can then be compared in the planning process or examined for the potential for improvement using AI.
- Satellite image evaluation: By processing satellite images, changes in the landscape, such as deforestation, traffic volume or the progress in construction projects, can be observed.
- Master data quality analysis: In addition to descriptive evaluation of the quality of master data, an AI-based identification of entries with poor quality can also be established. This allows better data storage and correction of errors.
- IT infrastructure usage: Whether to optimize the system landscape or in preparation for the conversion of systems, logging and ML can show dependencies between systems and their use.
- Data source combination: information on a product or service is usually distributed over many systems, and there is rarely a unique identifier for all sources. These data sources can be combined through flexible (fuzzy) matching, and thus holistic analyzes can be carried out on an entity.
Industry 4.0 (Manufacturing)
- Predictive Maintenance for Industry 4.0: The prediction of necessary maintenance of machines before they stop. The aim is to avoid damage, repairs and thus loss of money and time, but at the same time, in contrast to planned Maintenance, to avoid unnecessary downtime.
- Automated end of line testing / Quality assurance: By combining MES and ERP data, classifiers can be trained that carry out automated quality control after production. This can be based on images, sounds, sensors or purely data.
- Demand forecasting: similar to sales forecasting, but with a stronger focus on the supply chain. Orchestration of the flow of goods to cover emerging needs.
- Digital twin: The digital image of a real product or a customer is a major challenge. However, once you have taken this step, it allows for a large number of analyzes, from product security and user behaviour to efficiency optimization.
- Robotic Process Automation (RPA): RPAs can be used to automate and run repetitive tasks. Both on a software and hardware basis.
- “Lights out” factory: Like the fully automated warehouse, fully automated productions can save resources and thus costs in many areas.
- Employee hot spots: Hotspots in a production facility can be identified using movement tracking. This provides starting points for optimizing walking routes, guiding material trolleys or simply improving the interior design.
Internet of Things (IoT) / connected devices
- Predictive Maintenance for products: Creating a “wear and tear” of products to recommend Maintenance, repairs or spare parts. Uses data on the use of the product and user behaviour.
- Obstacle detection: If a product such as a car, a lawnmower or a vacuum cleaner moves through an unknown environment, it is important to recognize and observe obstacles as early as possible.
- Prediction of product usage: Many products have different modes used for different purposes, such as economy mode or high performance. The next application can be predicted using association analysis or similar algorithms, and a recommendation for the appropriate mode can be given.
- Product usage profile: Electronic devices can use pattern recognition to prepare for their use. For example, a refrigerator can cool down, an oven can preheat, or a heater can turn on.
- Environmental impact analysis: Whether it’s a car, household appliances, or other resource-polluting machines, the user’s type, duration, and environment can determine their impact on the environment.
- UI optimization: Using behaviour analysis and master data, an optimal UI can be played out for different segments within a target group.
- Product tracking: The location of devices is determined utilizing RFID or QR codes, in which the customers receive a benefit (e.g. extended warranty period). Above all, it helps companies in the B2B2C market get to know their customers’ customers.
- Wearables: The increased establishment of wearables / smart accessories will lead to a glut of data directly from the customer. Particularly interesting for the analysis of lifestyle habits, behaviour and personal well-being.
Banking, Insurance & Finance
- Fraud detection: high bookings, unusual activities at the end of the month or the lack of everyday actions – there are many tips for detecting and stopping attempted fraud. The use of big data enables an efficient review.
- Creditworthiness / Loan Risk: Instead of relying on rules or scoring systems when granting loans, more and more banks are switching to machine learning methods to include a range of variable factors.
- Credit Card Churn: When do we expect customers to cancel their credit cards? Analysis and prediction.
- Loan recommendation: Use consumption and savings behaviour to predict when and if a customer intends to take out a loan. Possible for transitional loans in small amounts or larger loans, for example, for buying a house.
- House value evaluation: AI-based evaluation of a property or house. Item value or lifespan in the market.
- Insurance Claim Anomalies: Detect and flag anomalies when submitting insurance claims, indicating the likelihood that the insurance claim is incorrect.
- Banknote validation: Identification of counterfeit banknotes, potentially directly at the counter or ATM when entering or exiting.
- Auto-Trader: Automated trading based on AI (often reinforcement learning). Of course, a kind of holy grail is the prediction of market movements and the corresponding triggering of actions such as the sale of shares.
- Automated rating: Classify companies into a rating category based on a large number of factors. It can be found in both unsupervised learning (e.g. clustering) and classification (e.g. neural networks).
- Debt Collection: Categorization of debtors and the corresponding probability of repayment. Recommendations for action and corresponding ROIs.