What Are The Deep Fakes, How Do They Work And What Are The Uses?
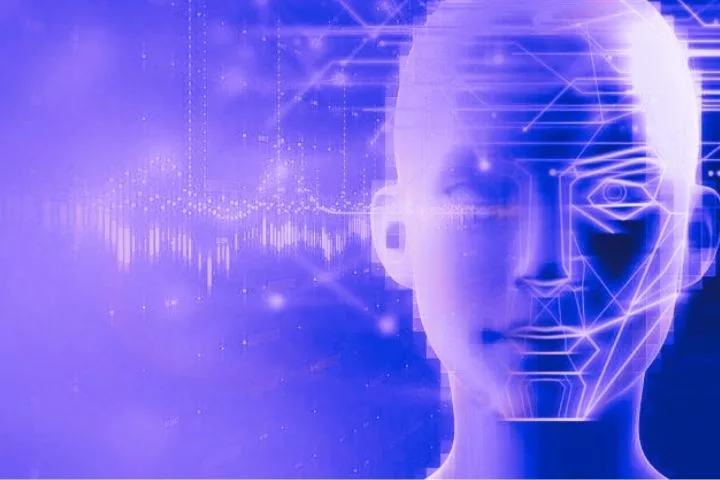
Deep fakes are artificially generated content (e.g., videos) that can hardly be distinguished from the natural material. Their creation is based on artificial intelligence, neural networks, and can create new versions of existing material with different people, languages , or content.
The risk of deep fakes is not insignificant: Counterfeit content can quickly cause damage to both private individuals and politicians. In this article, we discuss the methodology behind deep fakes, the danger, and the measures used against them.
What are deep fakes?
Deepfakes are videos, images, or even sounds artificially generated and have no basis for truth. The term “deepfakes,” in German “deep fakes,” is made up of the terms “deep learning,” a method in machine learning, and “fakes,” an art term for forgeries.
Deep learning is the technological basis for creating deep fakes, so artificial neural networks are used to create a so-called model from existing material (e.g., video material), generating new material.
Since the model is an abstract concept, this also means that completely new and unplanned material can be created.
The origin of deep fakes is autoencoders or Generative Adversarial Networks (GANs), which were conceptualized in 2013. It wasn’t until 2017 that the deepfake concept received wider attention, and the term became common.
While the general idea of media falsification is not new, deepfakes go far beyond conventional methods, as they make very complex and realistic fakes such as exchanging the voice or body possible.
How can you create deepfakes?
You only need basic material for deepfakes, from which the neural network can learn. These can be existing videos or images. The higher the amount of data ( big data ), the better because the algorithm can learn better based on more information.
However, since only a few want to deal with the programming of autoencoders, there are now several programs that make it possible to create deepfakes without much prior knowledge.
At the forefront are currently Chinese apps such as “Zao” or the commercial provider “Deepfakes web β.” In general, however, it is important to exercise caution. The hype surrounding deepfakes also creates many dodgy providers who exploit people’s curiosity and install viruses or the like.
What are the uses for deepfakes?
In general, deepfakes can not only be used for “fakes” but also have meaningful areas of application. The generation of content, if of correspondingly high quality, could bring about a change, especially in the field of media production.
For example, GANs are already being used as creative input for product design and development. Large companies such as Zalando also use generative networks to no longer have to photograph and care for their clothes in all colors but create them quickly and easily using machine learning.
But there are also numerous ideas for deepfakes in particular. For example, the “post-filming” in film production could be realized using deepfakes instead of laboriously creating all actors on rebuilt sets.
But I find the thought that films might soon be fully personalized even more interesting. The plot stays the same, but the actors can be exchanged as desired.
What is the danger of deepfakes?
But in addition to this positive view, deepfakes in the status quo initially pose a considerable risk. You can only see that the first main area of application was formed: the creation of fake pornography.
Many celebrities have now been “projected” into dubious films to correspond visually to some people’s ideas. Applied to the private sector, this can quickly become a general problem if suddenly nude pictures or porn appear of people who have nothing to do with them.
In such a case, the effort to deny and the attempt to prosecute are, of course, in no relation to the simplicity of how such deepfakes can be produced and distributed.
While private individuals would rather bear the damage in these cases, there is also no risk to society as a whole with deepfakes. So far, fake news can only be found in writing, but deep learning, videos, or audio recordings will soon make the rounds that look deceptively real.
A simple example would be Angela Merkel’s announcement of protective tariffs, border closings, or even a declaration of war. Even if it could be identified in the long term that these are fakes, the initial damage is inconceivable.
And the worst thing is, in the end, reversing the problem. If in the future we live in a world in which everything can be a deepfake, many people will have problems distinguishing reality from it.
It can happen that people would dismiss real things as fake, which concern them very well, or believe other things, although they are fake. This opaqueness of truth will, therefore, not only have social, economic, and societal effects but also clearly psychologically.
What measures are there against deep fakes?
As you can see, deepfakes can cause serious problems. Roughly, there are three main thrusts in responding to deepfakes: legal, detection, and dissemination.
The first point is to ensure that those who create and distribute deepfakes can be held accountable. The guidelines and the corresponding penalties vary greatly: The USA, for example, explicitly prohibits deepfakes regarding public figures or fakes with sexual representations.
On the other hand, China requires deepfakes to be labeled – otherwise, there will be severe penalties for distributors and platforms alike. Other countries like Canada and the United Kingdom have less specific rules, but deepfakes are equally prosecutable.
The second approach is the detection of deepfakes using other algorithms. For this purpose, simple inconsistencies such as incorrect brightness or shadows are detected in videos and thus marked as fakes.
Of course, deep learning is also used here. While the idea of having algorithms fight against algorithms is very futuristic and interesting, this approach resembles a technological arms race.
Deepfake algorithms can continuously improve by using the deepfake detection algorithms to correct their errors, which requires action on the part of the detection.
The third approach is a systematic exclusion of dissemination, utilizing a prohibition through terms of use on platforms. Reddit, Twitter, Discord, and Vice are just a few platforms that now partially exclude deepfakes and block disseminating users. Since this approach is also based on detection, this is not a definitive solution either.
We would like to add knowledge transfer as a fourth, often disregarded and therefore hardly discussed thrust. Promote awareness of the methods, possibilities, and thus the existence of deepfakes.
You can also ensure that the responsible citizen is more careful with both their material (for training) and foreign material (as human detection). So if you manage to increase the digital competence of Internet users and establish the principle of “don’t believe everything,” the damage caused by deepfakes can be limited better than with other methods.