What Is Smart Data? Definition And explanation of the term
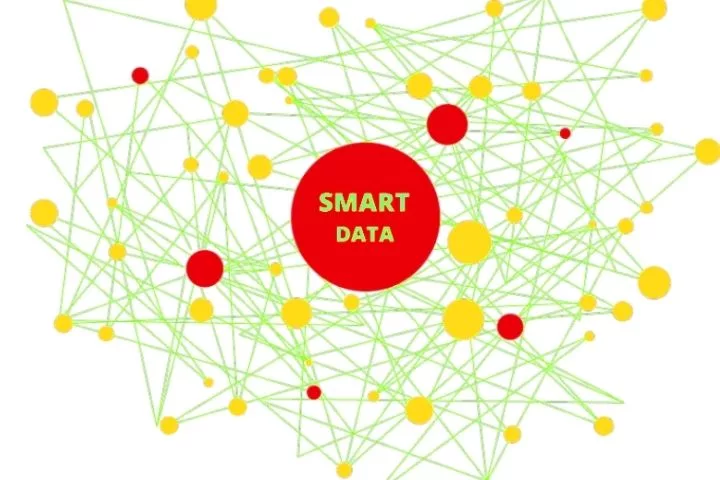
Data sets that have been prepared for direct and efficient use are referred to as smart data. As a result, it has already been consolidated, checked for quality, and, ideally, processed with meaningful analyses.
As a concept, demonstrates the issue in today’s corporate world: There is a lot of data, and it is becoming more accessible as the digital transformation progresses.
However, there are numerous other obstacles, ranging from data quality and reliable processing to provision via sustainable infrastructure.
These aspects are not immediately apparent in technologies like data science, big data, and machine learning – but when a company deals with advanced analytics, the challenges become apparent.
Why is it so valuable?
As previously stated, the database begins to establish itself as the digital transformation progresses. However, because of the sheer volume of data in a company, combined with the lowest processing capacities, the knowledge contained within it is frequently wasted.
This valuable “oil” is inaccessible to the average worker. It necessitates the use of specialists such as data scientists.
And this is the crux of the problem: businesses have a lot of data but aren’t given the tools to use it. As a result, all data sets classified as “smart data” are valuable to the company, whereas raw data is frequently a burden.
As a result, most businesses value data only when it is “smart data.” They will only be useful if they are usable and understandable for normal users. Do they add value to the organization?
The smart data process is visualized.
The process of transforming data into smart data follows six steps, which primarily integrate processes from data engineering, data governance and data science:
- Data acquisition: Whether from external or internal sources or the generation of new data – the first thing to do is to generate and acquire data.
- Data consolidation: Partly optional, but usually, individual data sources are linked to one another and brought to the same level of granularity to provide a uniform picture.
- Data quality analysis: Once the data is available in its raw format, the first thing to do is check the data quality and correct it if necessary.
- Data aggregation: If the raw data is of high quality, it is usually brought into an aggregated format. This is useful for visualization and the preparation of machine learning or other algorithms as feature engineering.
- Data evaluation: Whether classic data analysis or advanced analytics with artificial intelligence – the processing of the data and the conversion into meaningful information is at the centre of transforming raw data into smart data.
- Data provision: The last important aspect is often overlooked. Because it is not enough to create a great machine learning model, the analyzes and models must also be used. The operationalization concludes and makes the resulting data available to the relevant stakeholders for use.
What’s the difference between smart data and large data?
Smart data is frequently portrayed as a stage in the growth of big da
ta. The link is that the hype around big data has raised the general data potential and perception of data utilization. However, in practice, you rapidly experience several obstacles, which is why smart data is designated as the next level.
The link is so obvious, but it is not true that it can only emerge from huge data. Any data may be classified as smart data if it is adequately prepared and adds value. As a result, Big Data does not own the concept of “smart data.”
What’s the difference between smart data and advanced analytics?
There are several types of advanced analytics, including artificial intelligence, machine learning, prediction, and prescriptive analytics.
Smart data, like big data, is strongly related to advanced analytics, although it is not the sole use case. Traditional descriptive assessments can also have a significant influence, provided they are appropriately suited to the demands of the stakeholders. As a result, advanced analytics is frequently one data processing method, but not the only one.
The role of “smart data” in the data-driven company
While smart data as a term is certainly a good way to represent the difference between existing and processed data, for us, it is just a trailblazer.
The goal must not be to categorize data into “Smart” and “Dumb”, but rather to establish the holistic image of the Data-Driven Company.
It is, therefore, important to convey that the mere existence of data does not provide any value but that a lot of work is required to process it. But there is much more to understand how far-reaching the effort is.