Artificial Intelligence: Working, Procedure Models And Use Cases
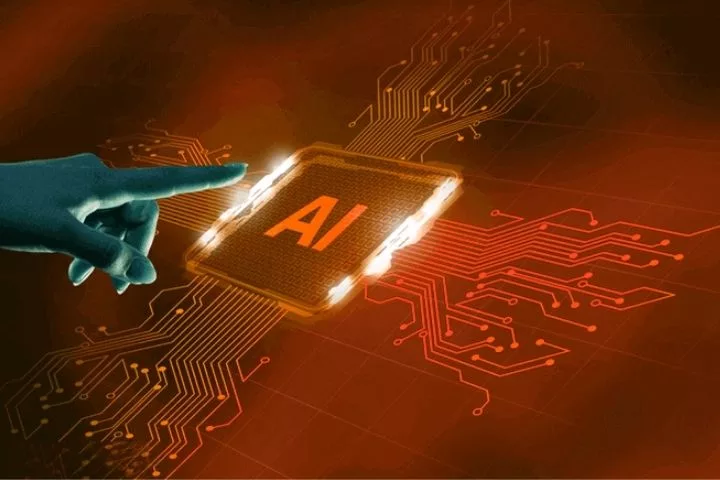
You hear about artificial intelligence (AI) everywhere. Often the term does not seem tangible. What do the terms related to AI mean? How does an AI system work? We have summarized for you what is behind it.
Artificial intelligence (KI, also AI for Artificial Intelligence): Artificial intelligence describes technologies that imitate cognitive competencies that only humans were previously capable of.
This includes, for example, strategic thinking or language skills. In this way, employees can often be supported in repetitive and time-consuming activities that previously required a lot of attention.
AI that is used today solves specific problems in a firmly described setting with methods “that are developed and optimized for the respective requirements” (so-called weak AI / narrow AI) and do not attempt To imitate people (so-called strong AI). The preoccupation with artificial intelligence goes back to the 1930s,
Machine Learning (ML): Machine learning is a sub-area of Artificial Intelligence. It is often used synonymously with AI, but it only represents an essential sub-area in which relationships from data can be learned independently. There are three types:
- In supervised learning, an algorithm is “fed” with exemplary inputs and outputs. By comparing the algorithm’s results with the accurate outcomes, the method learns to also predict outputs for unknown inputs (e.g., classification of new inputs).
- Unsupervised learning aims to recognize patterns in data. To do this, an algorithm receives inputs and uses them to create a model that depicts the internal relationships (e.g., similarities).
- In reinforcement learning, an algorithm learns through reward and punishment (numerical values such as the score of a computer game) which actions make the most sense in which state to achieve a specific goal.
Deep Learning (DL, German: multi-layered / deep learning): Deep learning is a method of machine learning in which an algorithm learns to recognize or map relationships in vast amounts of data with the help of artificial neural networks.
Artificial neural networks: These are based on the human brain to map complex relationships similarly. Individual neurons perform simple operations. If these are linked in hundreds, thousands, or more, complicated relationships can be learned and mapped together.
This is how it works:
A kind of AI system is to be considered as a so-called software agent. This perceives (like humans) certain aspects of the environment and, in turn, affects them (see illustration).
The difference to humans: The AI system is fed by sensors in machines, ERP systems, customer relationship management systems, and even Internet data. Using mathematical and IT methods, AI systems can then fulfill the following functions:
- Assessment of perceptions
- Deriving further conclusions
- Derivation and execution of actions Where can artificial intelligence be used? Business areas and industrial use cases
Where can artificial intelligence be used? Business areas and industrial use cases?
There are innumerable possible uses for artificial intelligence. But what does that mean in concrete terms? We will show you examples of potential benefits in the industry for various company areas – from sales to quality assurance.
The term “Artificial Intelligence” (AI) was explained and made more tangible in the previous post. The next step is about the specific benefits of AI for different areas and use cases.
In principle, the use cases can be classified according to the already described functions assess – deduce – react.
When judging, it comes to infer the state of the environment or the observed object from data. Where many handwritten documents are received in order acceptance, automatic scanning, for example, can save staff a lot of time.
In production, it is also difficult as a human being to recognize critical developments in values from a mass of real-time data – machine learning processes help with this assessment.
AI applications with a focus on reasoning offer employees new insights and additional information from data. In this way, data from the past can be evaluated to create forecasts for future value developments or to generate proposed solutions.
The actual decision about the appropriate actions, however, is made by the people. Predictive maintenance describes z. B. Applications that use past sensor values and related machine failures to calculate when failures are expected.
Care can thus be optimized. A forecast of sales figures can also help to adapt the purchasing figures to future demand.
For AI systems to be able to react, they need direct interaction with the environment. B. from experience and the respective success or influence of their actions.
In other use cases, judgment functions are used to then carry out the respective actions based on algorithms. Driverless transport systems (AGV), for example, transport components between the warehouse and production halls, while robotics process automation (RPA) analyzes and automates repetitive computer work.
The examples make it clear: Today’s artificial intelligence does not replace people but rather individual skills. AI systems do not come close to abilities inherent in humans – such as deriving actions in complex or rare cases.
In addition, technical implementation options are only one side: the organizational challenges such as social habits, fears, and employee participation requirements must also be considered. Always a good tip: get employees on board early!
How can the potential and feasibility of AI be identified? Procedure models and criteria?
Artificial intelligence (AI) can be used in a variety of ways. How to identify potential and feasibility in your own company and which procedure you can use to orient yourself when implementing AI projects is presented here.
The previous contributions explained how AI works and presented possible applications in companies. Now it’s all about target-oriented use: On the one hand, it is essential to identify and evaluate potential, and on the other hand, AI projects need to be precisely planned and implemented.
Identify potential and feasibility.
To identify suitable AI use cases in your own company at a workshop, four phases have proven themselves:
In the 1) preparation, who is there is clarified. Management and executives are, of course, critical.
However, employees who know specific company processes well through their everyday work and will later use the AI applications – whether in the company itself or at suppliers or customers, are also indispensable.
During the preparation, the parties involved must ensure a common basic understanding of AI to identify approaches in their own company.
For the 2) brainstorming and prioritization of ideas, there are many other methods to come up with good suggestions in the group and filter them. Openness and respect for the knowledge and experience of the other participants are elementary here.
In the subsequent 3) elaboration of the use cases and quantifiable benefits such as reduced machine downtime, soft factors such as internal competence development also play a role.
Like a business model canvas for evaluating business models, a structured consideration of individual aspects such as data availability and possible AI-supported actions can help specifically work out the use cases and present them.
Finally, the 4) evaluation & decision of the use cases are pending. In addition to the set-up know-how, the competence with which the AI system can be continuously operated and adapted to changing processes (so-called machine learning operations, MLOps) must also be considered.
Based on these factors, either a make, buy or wait decision (for applications with a high level of research and development dynamics) can be made.
Since all AI systems are based on existing data, data availability, volume, and quality are central to assessing feasibility and project success.
Domain experts from the respective company division can best evaluate the representativeness of the available data. Legal and ethical limits must also be complied with, such as personal data and AI decisions that can influence employees. The European Commission’s “Assessment List for Trustworthy AI” helps.
Successfully plan and implement AI projects.
With a use case, project budget, project team, and the intra-structure, the implementation then begins. Eight phases are run through partially iteratively:
- Everyday business and project understanding: All those involved specify the requirements, framework conditions, and success criteria in a project plan.
- Understanding technology, processes, and data: Technology experts in the company, in particular, provide AI experts with the knowledge they need to classify the data. This includes information about the cause of errors or outliers or the meaning of the individual data.
- Data preparation: AI experts: select, clean, filter, and format the raw data to identify and construct meaningful features for data-based modeling. This can sometimes take up the longest time in the project.
- Modeling: Here, suitable algorithms are selected, and models derive the desired findings from the available data.
- Evaluation: To assess the added value, whether the success criteria, requirements, and business goals are achieved or whether a new development cycle has to be entered into.
- Provision: In the best-case scenario, the user-friendly and operational solution is embedded in existing systems and platforms; users must also be trained in operation and interventions.
- Further development through continuous data collection: The model’s accuracy can be increased or further developed from the constant supply of data and the parameters identified from it and, if necessary, data sources.
- Maintenance: The AI model is continuously monitored and retrained by MLOps engineers (machine learning operations).