Know About The Ingrowing Demand Of Natural Language Processing
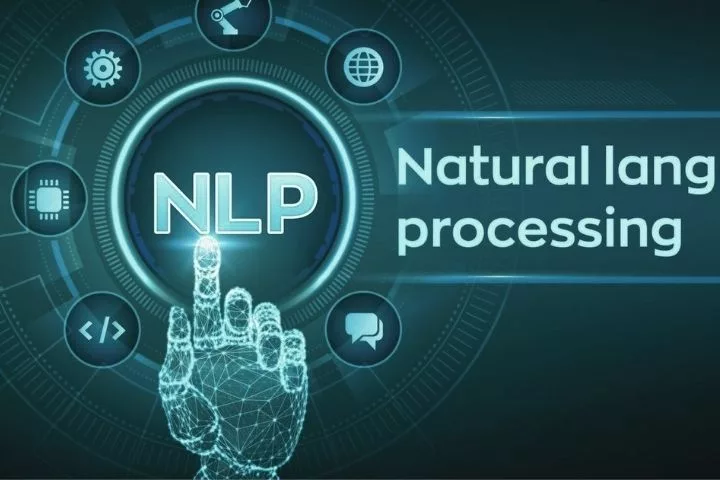
Among the areas in which artificial intelligence techniques for NLP are producing more satisfactory results, we find intelligent conversational systems.
These systems – among the most famous, Amazon’s Alexa and Apple’s Siri – are able not only to imitate human dialogue but also to answer questions on topics of different nature (from the latest news to movies on TV) and to perform complex tasks (such as planning a trip).
Significant progress has been made in this field, and meaningful results have been achieved, mainly thanks to deep learning algorithms, but there are still several open research challenges. Let’s see how to deal with them, but before delving into them, let’s reconstruct the birth and evolution of NLP techniques.
Social networks and big data at the basis of the progress of Natural Language Processing
From the birth of the Internet and up to 2003, the year in which the first social networks such as Facebook and Linkedin appeared on the international scene, a few dozen exabytes of data were available on the Web.
Today, that same amount of data is created weekly. This phenomenon of massive generation and dissemination of information is attributable to various factors.
First of all, the advent of the Social Web and the availability of pervasive technological devices (smartphones, tablets) have changed the characteristics of the average user, who, from being a passive and occasional user of information mainly disseminated by institutional sources, has become an active protagonist.
And constantly operational, increasingly involved in the production of its content, in modifying other people’s content and in activities relating to the purchase and sale of objects and services.
This has led to the generation of an incredible amount of textual data of a highly heterogeneous nature, expressed in different languages and formats.
Secondly, the computerization of business processes and document digitization have led to a continuous and exponential increase in data, for the most part textual, produced and held by public administrations, hospitals, banks, law firms, private companies.
The meeting between Natural Language Processing and Deep Learning
In this context, characterized by an extreme variety and quantity of contents expressed in natural language, the use of artificial intelligence assumes strategic importance, favouring the creation of innovative solutions for processing, understanding and production automatically.
Of textual data. In particular, in recent years, we have witnessed the emergence of new approaches that integrate natural language processing with deep learning algorithms ( deep learning ), producing extraordinary results in different application scenarios.
Thanks to them, it is now possible to automatically translate texts or speech between different languages with surprising performances, to dialogue and ask questions to machines in natural language on specific domains, to extract relevant knowledge and insights, with both informative and predictive value. , from vast amounts of textual data, to generate natural language content, for example, to synthesize essential information from one or more documents, or to determine the polarity of text that contains opinions, for example, about products, services, individuals, events.
What is Natural Language Processing
Natural Language Processing is an interdisciplinary research field that embraces computer science, artificial intelligence and linguistics, to develop algorithms capable of analyzing, representing and therefore “understanding” natural language, written or spoken, in a similar or even more performing than humans.
This “understanding” is determined by understanding, and therefore being able to use, the language at various granularities, from words, about their meaning and appropriateness of use concerning a context, to grammar and rules of structuring both sentences starting from words and paragraphs and pages starting from penalties.
In greater detail, first of all, NLP provides solutions to analyze the syntactic structure of the text, associating the respective morphological categories to the individual words (e.g. noun, verb, adjective), identifying entities and classifying them into predefined categories (e.g. . person, date, place), extracting syntactic dependencies (e.g. subjects and complements) and semantic relationships (e.g. hyperonymy, meronymy).
Secondly, it allows you to understand the semantics of the text, identifying the meaning of words, also related to the context and methods of use (e.g. irony, sarcasm, sentiment, mood), classifying it into predefined categories (e.g. sport, geography, medicine) or summarizing its content.
Despite the convincing results obtained in different applications, for example, with search engines and in the extraction of knowledge, the need to further improve the intuitive understanding of natural language content, reaching levels similar to those of the human being, represents, still, an open challenge on which the research world is working.
Deep learning for natural language processing and understanding
In 2011, for the first time, a simple algorithm based on deep learning was applied to different NLP problems, including the identification of entities and the assignment of morphological categories to words, showing significantly better performance than other approaches representative of state of the art.
Since then, more complex algorithms based on deep learning have been developed to tackle NLP problems not yet solved or treated in the past but with unsatisfactory results.
Deep learning, in short, is based on the concept of the artificial neural network, that is, a mathematical model inspired, from a functional point of view, by the biological neural systems of the human brain.
A deep artificial neural network comprises a series of neurons that are arranged on multiple levels connected. one of important technology
A first fundamental characteristic of these networks is that they can learn, autonomously and contextually, both a hierarchical representation of the best descriptive elements of the input data (not necessarily intelligible by the human being) and how to combine this information in the best possible way to solve a specific task.
A second important feature is that such networks, similar to the human brain, can learn from their experiences, that is, to improve their performance in solving a complex problem according to the number of examples with which they are trained.
However, these networks can process, as input, only numeric data and not textual strings. This is one of the reasons why the first successful applications of deep learning involved the processing of images or signals.
To date, deep learning, through the combination of word embeddings and convolutional and recurrent networks, represents the most adopted approach to address problems related to the processing and understanding of natural language , not only in the academic field but also, and above all, in industry, taking the form of products and applications, now in daily use, released by multinational companies such as Microsoft, Google, Facebook and Amazon.
NLP in conversational systems
One of the research challenges in which deep learning-based NLP techniques are finding more and more application concerns the development of intelligent conversational systems, able to emulate human dialogue, answer questions on different topics and perform complex tasks.
They also offer highly heterogeneous advanced functionalities, with promising results both in the world of research and in industry. Extremely well-known examples of such systems are Microsoft’s Cortana, Amazon’s Alexa, Apple’s Siri, and Google’s Assistant.
Supporting paraphrases and favouring semantic inferences.
They can then “chat” naturally with humans, providing support, assistance and recommendations ( generative chatbots ). This is made possible thanks to deep learning algorithms similar to those used in automatic translation systems, which make it possible to learn different types of answers on open domains starting from examples of dialogue (“translating” each question into an answer).
Finally, they support the user in carrying out more or less complex tasks, ranging from organizing a meeting to planning a vacation ( task-oriented conversational systems ).
To do this, they use deep learning solutions trained to understand the user’s intentions, expressed through requests formulated in natural language, update the status of the conversation according to these intentions, select the next action to be performed and convert it into an answer also expressed in natural language.
These are just some examples of the possible functionalities of the latest generation conversational systems created using NLP techniques.
The enormous progress achieved in the field has been achieved only thanks to the use of deep learning algorithms and the availability of large amounts of data to be used for their training, with reference, for example, to the classification of questions or the articulation of dialogues.
The new challenges for the NLP
Despite the enormous progress and countless results recently obtained in the field of NLP, mainly thanks to the application of deep learning algorithms, there are still several open research challenges, which can be summarized as follows.
- Automatic natural language processing for languages or application scenarios with limited availability of annotated data. Deep learning approaches, for example, to classify or assign polarity to a text, use a supervised learning model, i.e. they require training datasets labelled with the classes or phenomena to be determined (the topic or polarity). Annotating datasets is an intensive process that requires time and human resources to complete. While for the most widely used languages, such as English, or widely used application scenarios, such as sentiment analysis, there is wider availability of annotated datasets and, therefore, a greater possibility of training performing deep learning models for many other languages or scenarios there is a lack of usable data for this purpose. Currently, different approaches are being studied to deal with this problem,
- From a more specifically cognitive perspective, understanding natural language in human beings does not occur in isolation, without any information on the context and the surrounding environment. Still, it is closely related to perceptual experience and sensorimotor interactions with the external world. In light of this, reproducing in machines the ability to understand and produce the language of the human being cannot ignore the creation and correlation of conceptual and sensorimotor representations of objects in the surrounding environment. For this purpose, currently,
- By common sense reasoning, we mean, for example, those that allow us to understand who a pronoun refers to in a sentence or that the penguin does not fly, despite being a bird and birds do fly. To date, despite several advances made, teaching a machine to do common sense reasoning remains an unsolved problem. On the other hand, the absence of common sense in machines represents one of the barriers that, to date, have limited their ability both to understand the phenomena that occur in the surrounding world and to communicate naturally with human beings, not allowing them to behave reasonably in unknown situations and to learn from new experiences not contemplated in the training phase. Lately, to represent, understand and generate text or speech in natural language in a similar way to humans, and on the other hand, in having large amounts of data available, even aligned between different languages, to train and validate their performance.