Examples For Big Data & Machine Learning Use Cases In Healthcare And Customer Service
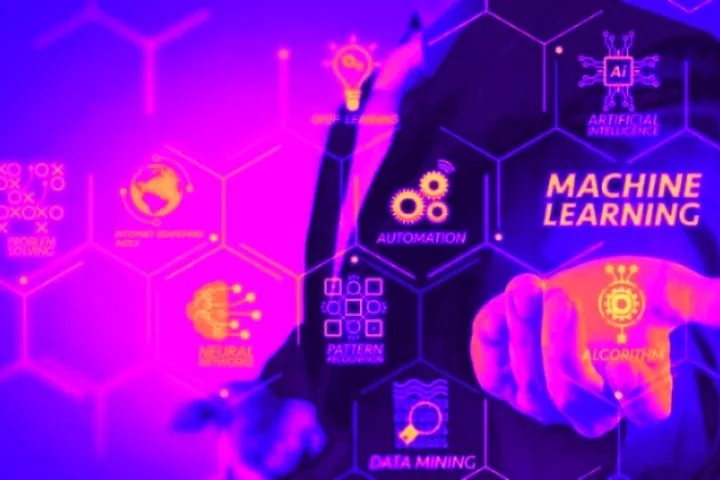
There is often a lack of examples of how technologies like artificial intelligence, big data, data science and machine learning can be implemented as use cases. We have therefore compiled a list of Big Data & Machine Learning Use Cases, which we are continuously expanding.
General Big Data & Machine Learning Use Cases in everyday life
- Text prediction: In the meantime, the prediction of sentence components is used in many areas. It is now standard, especially in the area of e-mail and mobile phones.
- Virtual Assistants: Siri, Alexa & Co are based on natural language processing and learning through their use. The amounts of data have to be transferred quickly and processed using machine learning.
- Face Recognition: Extraction and recognition of faces using image processing and classification. They are used in a state context but also as a security measure.
- Avatar generation: Creation of lifelike avatars based on image material without this person existing. This approach is already advanced in the field of images and has strong development in video.
- Crime prediction: Predicting crimes based on past crimes, population, season and other factors. Allows increased presence in the identified area at the identified time.
- Deep fakes: Using existing video or audio material, a generative, i.e. producing, a neural network is trained that produces similar material. So you can create real videos that are fake. This example is currently one of the biggest current ethical problems in machine learning.
- Automated devices: Vacuum cleaners and lawnmowers are just the beginning. More and more autonomous robots will populate our houses and need a variety of machine learning and cognitive computing algorithms for this.
- Recipe refinement: Automatic recognition of suggestions for improving/changing recipes (e.g., comments) and adapting them accordingly.
- Similar restaurants: Similar restaurants can be found and recommended automatically based on location, price and menu—association analysis, collaborative filtering or classification.
- Recognition of plants: Based on a photo of leaves or flowers, the type of plant is recognized, and information is displayed, ideally in combination with care tips and common pest control.
- Traffic control: Using predictive analytics and machine learning, traffic flows are predicted and controlled based on real developments, for example, through traffic lights or additional lanes. The goal is a higher flow rate and avoidance of traffic jams and thus avoidance of environmental pollution.
Healthcare, Sports & Fitness
- Individual setting of fitness equipment: Personalized seat and weight setting using stored data, a combination of different data sources (weight, performance, food …) and prediction of exercise type, intensity and duration.
- Medication intake: Prediction of the likelihood of whether patients will take their medication based on demographic factors, duration and type of medication and medical history. Allows the direct establishment of measures to ensure that they are taken.
- (Breast) cancer detection: Radiologists have the task of deciding on x-rays whether or not cancer is present. This can be supported or even replaced utilizing image recognition and machine learning.
- Drug creation: Creation of drugs based on Artificial Intelligence.
- Team composition: Predicting the best team for the opponent. Consideration of individual strengths and team synergies.
- Trading & Drafting Prediction: Prediction of the performance of athletes in team sports to support decisions in player trading.
- Injury Prediction & Prevention: Predict injuries and take appropriate countermeasures.
- Health monitoring: Smart devices such as fitness wristbands will make it easier and easier for patients to be at home and active again faster. To prevent dangers, these IoT devices can be enriched with AI to react proactively to changes in the state.
- Patient prioritization: patient care can be prioritized using patient data and historical disease progression. The aim is to lower the mortality rate and reduce occupancy.
Customer service, service & support
- Replacement part recognition: Image recognition and classification allow customers to suggest suitable products when looking for spare parts directly. Interesting for B2C and B2B alike.
- Chatbots: Whether B2B or B2C – chatbots have been one of the best methods for some years to relieve customer support and be available to customers around the clock with solutions and information.
- Incoming call classification: Categorization of callers and their concerns on the corresponding customer service teams or support level.
- Automated ticket answering: Responses from service employees are automatically pre-formulated and, if necessary, referenced to standard documentation such as FAQs.
- Repair cost proposal: Based on previous data on the acceptance of repair proposals and their efficiency, repair cost proposals can be developed automatically.
- Seasonal forecasts for service requirements: In many companies, there are seasonal fluctuations in the use of their products (sports, home improvement, garden). This information can be incorporated to prepare appropriate capacities in support functions.
- Service inquiries as product development: Through text analysis of service inquiries, important topics and negative aspects of products and services can be identified and thus improved.
- Prediction of service technician demand: Prediction of demand to plan technician deployment. Consideration of sales, durability, season, weather and much more.
Logistics, warehousing & supply chain
- Delivery routing : A classic topic, but with the support of AI a very efficient approach, as it can also easily include other factors such as traffic volume or priority.
- Logistics tracking : A sensible approach for internal efficiency analyzes on the one hand, and for the customer experience on the other. Generates very large amounts of data that have to be processed in real-time.
- Stock level alerts : Prediction of changes in stockkeeping requirements due to changes in demand. Alarms and notification so that critical changes are detected.
- Retail replenishment : By using distribution algorithms , it is possible to react fully automatically to retail requirements by orchestrating distribution centers. This can be reflected in rolling data (ramp-up over the course of the day) or peaks (sell-out).
- Fully automated warehouse : By means of automated path finding and image processing / machine vision (e.g. barcodes, surroundings, running lines), autonomously acting robots can take over both picking and packaging.
- Peak hour prediction : Prediction of workload and corresponding distribution on pre-occurrence time. Alternatively, appropriate workforce management to handle peaks.