Data Quality: Definition, Importance And Problems Of Poor Data Quality
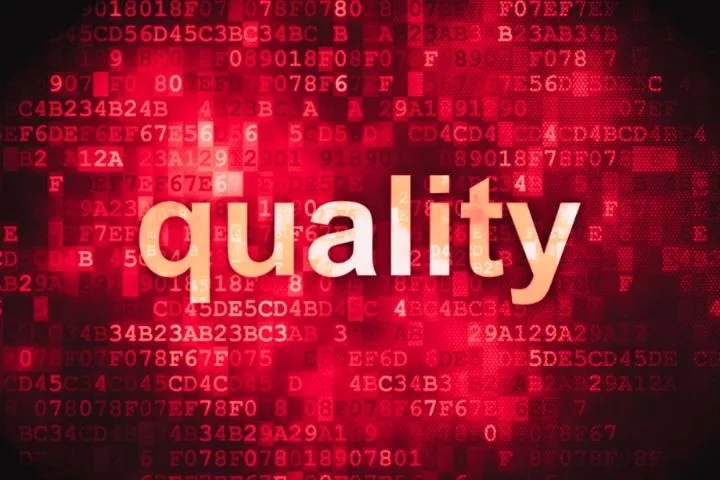
Data quality is becoming more and more important in companies. On the one hand, many problems are caused by poor data quality. On the other hand, high data quality offers a multitude of advantages for companies that want to work with the data.
Definition:
The point at which it arises is usually when data is to be used, and one of the participants notices that something is wrong. This “not correct” is intentionally formulated so vaguely because the manifestation of low data quality can be determined in many aspects: From missing data to duplicates (duplicates) to incorrectly recorded data, there are many manifestations of poor data quality.
When we talk about it in a company, we usually mean the specific content and accuracy of detailed data.
How can data quality be classified in the subject of data governance and data management?
It is a topic in data management, which is the operational application of data governance. The difference is that data governance defines the goals, processes, organization, and guidelines for data management, while data management implements these framework conditions.
The final distinction is based on the fact that it is often only related to the structure and content of the data but not to the quality of the data process. However, this topic is the basis for all activities in data management, so it must be considered in the quality of the data.
Problems caused by poor data quality
Pretty much every company has had negative experiences with poor data quality. This is not surprising: data is applied to pretty much every company process, and poor quality leads to a poor result within the process. Here is a list of problems that can arise.
INCREASED COSTS DUE TO THE ADDITIONAL EFFORT
Whether it is for customers or suppliers, poor master data quality can lead to misspelled offers, service employees to the wrong address, or deliveries to the wrong places. It is therefore increasingly crucial for operations that all company data is as correct as possible.
WRONG ANALYZES
With the increase in data-based work, data analysis, and data science use cases, the importance of data quality also increases. Incorrect analyzes or incorrect machine learning modeling quickly lead to wrong decisions being made. And this quickly costs money: Regardless of whether it is lower effects, incorrect strategic choices, or incorrect monitoring of business processes
PROBLEMS WITH THE IMPLEMENTATION OF LEGAL REQUIREMENTS (COMPLIANCE)
While many points are about efficiency, lost sales, or the like, legal situations quickly become business-threatening. Especially in times of GDPR and the right to be forgotten, missing data governance, incorrect links, or missing documentation quickly becomes a far-reaching problem.
LOSS OF SALES
Another problem is a direct impact on (potential) sales. Particularly in the area of master data, care is required here. Suppose proper lead management is not carried out.
In that case, customer data is not maintained, or the quality of transaction data is insufficient to analyze product recommendations or segmentation, sales are quickly lost.
BAD DATA CAN DAMAGE YOUR IMAGE
One last but important topic that many are often not directly aware of: The image of a company can also suffer greatly from poor data quality. For example, if the quality of the master data is low, incorrect product information may be communicated to the customer via the channels. This leads to confusion or frustration when receiving different products than expected.
Another example is in the area of user experience. If customers expect delivery based on incorrect or out-of-date data, the customer will have a terrible experience.
How lousy data quality arises in a company
BAD DOCUMENTATION OF DATA
In the absence of data governance, it is unclear when, how, and where data can or should be documented. As a result, it is unclear what data is available, what attributes it has, or how it is used.
This leads directly to poor quality on the one hand but the inferior quality of the overall process on the other. Therefore, poor documentation of target data and the existing content is usually right at the center of poor data quality.
DATA SILOS
Due to the historical structure of data silos, there was never a need to use the data for other purposes. Neither connect across systems (data unification) nor collect centrally for analysis (e.g., via data lake ).
Due to this lack of use and data silos, the focus was never on quality, as data is often better maintained within a system than across systems and purposes. Gradually, these legacy systems will be replaced, and the linking of silos will become even more relevant.
UNCLEAR STRATEGY
If there is no clear data strategy, no vision can be conveyed in the company. As a result, essential work such as building a uniform data infrastructure, expertise in data engineering, or data governance is rarely a priority.
NO DATA GOVERNANCE
The organizational and strategic lack of a data governance initiative is fundamental to the continued low quality. Data governance is the direct counteraction, so companies that have not established it also struggle more with poor quality data.
LACK OF COORDINATION BETWEEN TECHNOLOGY AND DOMAIN
Often there is simply a lack of precise coordination between the various technology departments to clearly define which data is most effective in which form.
HUMAN FAILURE
Depending on the study, humans are responsible for up to 60% of the errors in the data. Therefore, every company must set itself the goal of using processes, technological preventive measures, and transparent data governance principles to train and support its employees in making their contribution to high data quality.
How high data quality is defined and why it is so important
The value of high data quality takes many forms.
How exactly high is defined must be determined individually for each company. The consensus is that data quality is high when available and usable for the intended purpose. But this generic definition must, of course, be precisely defined using a tailored data governance program and underpinned by KPIs.
While the exact definition and its implementation in the company are individual, there are some general categories in which high data quality has a positive effect. These categories can be found in almost every company and are therefore considered a guideline, which is why it is worth investing in high-quality data.
CONFIDENCE IN THE DATA THROUGH BETTER DECISIONS, BETTER DECISIONS THROUGH CONFIDENCE IN THE DATA
It’s a cycle. You have a better starting position if you trust well-analyzed data (“data confidence”) and make decisions based on it. However, this only works if the data is trustworthy: If the underlying information is poor and you make a wrong decision based on this, this will, of course, destroy trust in the data.
Therefore, it is not surprising that 84% of managing directors have concerns about the quality of the data in their company – and accordingly only trust the data to a limited extent. And consequently, make your decisions again on gut instinct.
This cycle can only be broken if the quality of the is high. Because then you can use the data as the tool for which it is intended.
THE CONSISTENCY AND COHERENCE OF THE DATA ARE THE BASIS FOR WORKING TOGETHER
If data is interpreted differently for each evaluation, if different reports show different numbers, if other branches of the company are shown different stock levels – all these are factors that mean that a poor database destroys the basis for cooperation.
As a result, you can work together efficiently and stringently if you have the same basis in the data. And this is achieved through data governance, on the one hand, but also purely by increasing the data quality itself to minimize potential errors.
SYSTEM AND PRODUCT STABILITY
IT departments or exceptional data engineers are often busy repairing data pipelines after a change has been made or the data’s original format has changed.
In addition, there are often enough other problems with data that appear in a pipeline but are not expected. This type of data was not documented in the start system or because it does not correspond to the standard format – both aspects of low data quality.
In the worst case, such errors in data pipelines lead to the fact that downstream systems can no longer operate. Depending on the system, this leads to heavy losses in day-to-day business, which can be prevented through improved data quality.
USE DATA SCIENCE EXPERTISE IN A FOCUSED MANNER INSTEAD OF CLEANING UP DATA
In data analysis, the experts from data science and machine learning are busy looking for, cleaning, and preparing the available data 70% of the time. This is a time-consuming, frustrating task that can be wholly replaced by high data quality.
As a result, the expertise can be used in a much more targeted manner instead of data scientists dealing with it. In addition, a high data quality always allows a higher rate of the overall results – thus a double effect.
SAVE COSTS, EFFORT, AND TIME
As can already be seen from the various aspects, poor data quality costs a company money, effort, time, and reputation. Therefore, one can gain in all these aspects through a continuously high quality. Both direct costs such as downtime and indirect costs such as the effort to resolve problems or image losses must be considered here.
Overall, high quality means that you don’t have to deal with meta-topics. There are fewer delays in implementation because data is available directly, efficiently, and in high quality. Project budgets are generally less burdened because the data extractions are carried out without problems.
To quantify the effect minimally: According to a study by IBM in 2016, around 3.1 trillion US dollars are lost every year due to poor data quality.
COMPLIANCE READINESS: READY FOR DATA PROTECTION AND GDP
Defined above as one of the biggest problems, the problem with high quality can be defined as an advantage. If you have established a high data quality, this allows effortless compliance with legal requirements such as the “right to be forgotten” or delivering all stored data.
Since control over one’s data will shift more and more towards customers in the future, it is essential to be fundamentally prepared for the relevant legal requirements.
This includes precise traceability of existing data, documentation of the content, and simple cross-connection of all data sources in which customer data is located. And these are all issues for high data quality.
THE CUSTOMER AS THE BENEFICIARY
While many internal processes benefit significantly from high data quality, in the end, it is the customer who always helps. Whether it is more efficient or personalized channels, whether faster processing, better products, or services: everything pays off to have an advantage.
Therefore, data quality must not be viewed in isolation for internal tasks but must be thought through to the end of the process chain. Much of the information, whether master data or transaction data, ultimately impacts the channels and thus directly on the customer.
HIGH QUALITY LEADS TO HIGHER SALES
According to a study by Thomas Redman, companies miss around 15% to 25% of sales each year due to poor data quality. The logic is relatively simple: you can control all subsequent processes very reliably if you have reliable, validated data of high quality.
This direct influence is reflected in higher sales. Processes can be carried out more efficiently, customers can be better looked after, and orders can be fulfilled quickly. Not to mention personalized marketing and dynamic pricing policy. Everything leads to improvement of processes to minimize costs and better support for higher sales.
THE FUTURE IS DEFINED BY THE DATA – AND THE QUALITY HAS A BIG INFLUENCE
We firmly believe that the future belongs to data-driven companies. Those companies that actively record, store and use data will have a competitive advantage over companies that don’t.
However, since only the availability of data does not bring any advantage, but rather the ready-to-process provision and corresponding utilization, data quality is one of the main prerequisites on the way to becoming a data-driven company, alongside infrastructure, organization, and expertise.
Only then can a company go into the future-ready, scale its processes accordingly and defy the requirements of big data, data lakes, data science, and machine learning.