Big Data Veracity: What Is It? Definition, Examples And Summary
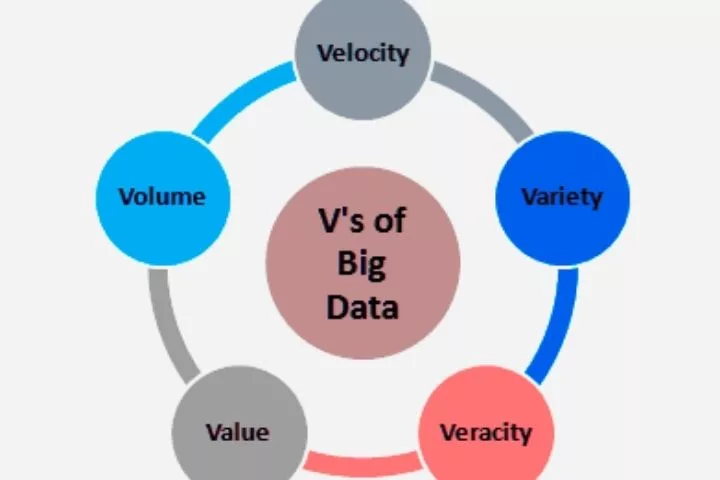
Veracity is an expression of the 5 Vs. of Big Data. But while the volume, velocity, variety, and value are relatively self-explanatory, big data veracity often raises questions.
Veracity stands for the (in) available data security: Can the data be trusted both in terms of origin and content?
The 5 Vs. of Big Data Definition
The five Vs. of Big Data evolved from the original 3 Vs. The name is also used as the initial definition of Big Data: the volume.
The volume of data plays a role. Sometimes, it was the first limiting factor: data could no longer be processed locally on a computer but had to be processed on servers or later distributed systems.
The second V is Velocity – the speed at which data is generated. This has two primary effects: on the one hand, the database to be analyzed changes continuously; on the other hand, this generated data must also be recorded in the system, which was or is not a given.
The third V is Variety, i.e., the variability of the data types. In addition to structured data (e.g., relational databases), unstructured data (e.g., images, audio, PDFs) are alluded to. Saving, documenting, and, above all, analyzing this data has raised new challenges.
The fourth V is the Veracity and was added to question the quality. We meet the more precise definition in the following section.
The fifth V is the value, i.e., the value of the recorded data. This has only to do with “big” data to a limited extent but applies comprehensively to all stored data. The company can only benefit from data that can be used sensibly.
The sixth V that was additionally recorded covers the variability of data. The variability is primarily aimed at data that change over time – such as seasonally changing data and their interpretation.
The fourth V: Big Data Veracity
In German, the Big Data Veracity, the “sincerity” or “truthfulness” of the data, deals with the quality of the available data. In particular, Veracity can be divided into two areas of origin and content.
The origin of the data is of great relevance so that the source’s trustworthiness can be defined. Internal records are usually more trustworthy than external records.
However, well-maintained data sets can contain a higher level of truth than neglected data sets. It is, therefore, always a difficult mental game to define which origin is the better.
Nevertheless, one would like to have this document – if only for that reason, if one wants to acquire newer, more, or different data on the same topic.
The more prominent part of Big Data Veracity, however, is the content of the data itself. The standardized term for this is data quality from the area of data governance.
As every data-driven company has recognized by now, data governance – i.e., the processes, principles, and implementation of data maintenance – is the next big challenge after data collection because neglected, uncleaned, or insufficiently defined and documented data usually only lead to one thing: poor analysis.
The “garbage in, garbage out” principle shows it most clearly. If you use poor quality data, no matter what effort you put into further processing and analysis, poor quality results are also expected. A company can only use data sensibly if this quality is prevented – i.e., if one dedicates oneself to the topic of Big Data Veracity.
In summary, one can see why “Veracity” made it onto the Big Data Vs. List. Not knowing where data comes from, not knowing how trustworthy the content is, and not knowing the quality of the data are indicators that you have to devote yourself to this topic separately.
Data governance initiatives that document and catalog data sources and define attributes are a good start; data stewardship programs for increasing data quality are a logical next step to becoming a data-driven company.
Receive further articles on the topic of Data-Driven companies directly
Examples of “veracious” data: Data veracity in practice
EXAMPLE 1: E-COMMERCE RAW DATA EXPORT FROM WEB ANALYTICS
When you export raw data from web analytics, there is often the problem that you cannot delete entries from external systems. For example, with Google Analytics, incorrect orders or bookings (e.g., due to bugs) are also exported. Inverted negative postings can only correct this if you know that you have to do this.
If one takes a raw data export and is unaware of this problem, it quickly distorts all analyses since incorrect postings are included. Likewise, to correct this, you first have to know which incorrect postings have to be cleared before you can restore the basic truth.
EXAMPLE 2: UNDOCUMENTED WEATHER DATA
In many search engines, weather data, either historical or live, are included as a factor. This data is rarely produced by the company itself but is almost always acquired externally.
If a company uses historical weather data neither original nor in content defined, problems quickly arise: Which weather stations are used? How do you get current data? If the service is switched off – how do we find an external service as similar as possible and that maps the same data basis? Classic examples of a lack of origin, content, and thus quality.
EXAMPLE 3: AGGREGATED SALES DATA IN THE DATA LAKE
One of the advantages of data lakes is the ability to hold both raw and processed data sets. If, for example, we access aggregated sales data, the calculation of which has not been documented, we quickly run into the problem of not assessing the origin or quality.
How was the data processed? Which booking types were selected? Were there filters? Many question marks should not arise for the correct and efficient analysis of data.
As a result, the data lake becomes a data swamp, and consumers cannot judge whether further data processing is useful or even wrong. All roles are required here, from data engineers to scientists to management, to guarantee high quality.
Summary of Big Data Veracity
If you look at these aspects together, the importance of Veracity in the Big Data context quickly becomes clear. It’s not just about knowing whether the content and thus the quality of the data is high, but also whether it comes from a trustworthy source and is suitable for further processing and interpretation.
It should not be forgotten that strategic, procedural, and operational decisions are based on data analysis. If these analyses are based on bad or incorrect data content, they can quickly lead to big problems.
We, therefore, recommend that you devote yourself to the subject of data truth, documentation, and quality in detail. Data governance, data management, and data stewardship are important topics in a data-driven company. A lot of time, energy, and budget must be invested to work in a future-oriented manner.